You need to understand the risks. Risks are perpetuating stereotypes, spreading misinformation, and security breaches.
Language models can introduce biased content and discriminatory outcomes. Ethical issues from algorithm design flaws. Data privacy breaches are big.
A good taxonomy helps you systematically identify and mitigate these risks, guiding the organization’s risk management efforts. Read on to learn more about managing the risks of language models.
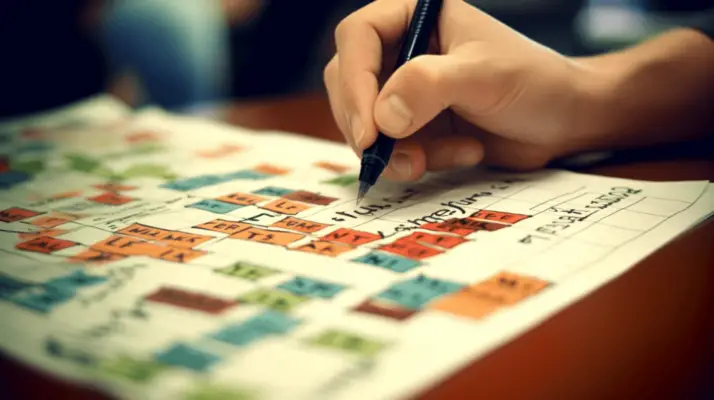
Takeaways
- Language model risks are biases, misinformation, and harmful stereotypes.
- Ethical risks from algorithmic biases and discriminatory outcomes.
- Security risks are data breaches and privacy violations.
- A taxonomy helps with targeted risk assessment and mitigation.
- Custom taxonomy for proactive risk management in language models.
Risk Taxonomy
Understanding risk taxonomy is key to identifying and addressing the risks of language models. Identifying risks within business processes is crucial for establishing a comprehensive risk taxonomy. By bucketing risks into areas like discrimination, misinformation, and exclusion, we get a clearer view of the problem.
This structured approach allows us to distinguish risk types, develop targeted mitigation strategies, and minimize harm and responsible model deployment.
Definition and Importance of Risk Taxonomy
A clear and structured risk taxonomy is essential for a comprehensive risk management approach. A risk taxonomy categorizes different types of risks so we can break down risks into specific forms. A well-defined risk taxonomy provides a framework to guide the organization’s risk management efforts in a structured and systematic way.
This hierarchical structure helps us to identify, assess, and manage risks consistently across business units and processes.
By using a risk taxonomy, organizations can prioritize and manage risks better and overall risk management practices. It is key in operational and financial risk, identification, internal audits, and managing identified and external risks.
Businesses can better understand and bucket risk events using a defined risk taxonomy and develop better risk mitigation strategies and a more robust operational framework.
Industry Use Cases for Risk Taxonomy
Industry use cases for risk taxonomy in language models are important for financial services and beyond, as they have unique challenges. Financial services must navigate operational risks around algorithm biases, data privacy, and regulatory compliance.
However, other industries have specific ethical and social implications when deploying language models and need customized risk assessments.
Financial Services and Operational Risk
Given the many risks in financial services, we must create custom risk taxonomies to identify and manage operational, credit risk, and market risks specific to this industry.
Financial institutions use risk taxonomies to the bucket and manage different types of risks, including operational risk appetite and risks from internal processes, people, and systems. Credit risk (borrowers not repaying loans) and market risk (fluctuations in financial markets) are also part of a comprehensive risk management framework.
Regulatory frameworks require implementing risk taxonomies to ensure compliance and robust risk management. The Chief Risk Officer oversees the development and implementation of risk taxonomies in financial institutions.
Beyond Financial Services: Industry-Specific Challenges
Across different industries, customized risk taxonomies help support us in identifying and managing industry-specific challenges.
In the domain of large language models (LLMs), industries face risks beyond financial services. The social risks of LLMs are amplifying harmful stereotypes and misinformation campaigns.
Ethical risks from biased algorithm design and discriminatory outcomes. Security risks like data breaches and privacy violations are big.
Creating an LLM taxonomy is key to risk evaluation and mitigation strategies for industry-specific challenges.
By understanding and addressing these risks upfront, we can navigate the complexity of deploying language models responsibly and avoid unintended consequences across different industries.
Building and Implementing a Risk Taxonomy
When building a risk taxonomy for language models, we must consider key components and best practices to the bucket and manage risks risk language used.
Overcoming these challenges is critical to the longevity of the risk taxonomy so we can do a proper risk assessment and management.
Key Components and Best Practices
Creating a risk taxonomy specific to your organization and industry is key to identifying and managing risks associated with language models.
A risk taxonomy should have key components such as risk categories, risk types, and risk events.
Best practices are to involve stakeholders actively in the process, use a hierarchical structure to bucket and classify risks by severity and likelihood, and review and update regularly to keep the taxonomy current and relevant.
Overcoming Challenges and Longevity
We must overcome challenges like thoroughness, stakeholder buy-in, and resource allocation to build a risk classification for language models.
Creating a detailed classification system that covers all types of risks from language models can be time-consuming and resource-heavy. Getting buy-in from stakeholders and ensuring the classification system is used consistently across the organization is key to its effectiveness.
Maintaining a classification system over time requires dedication and resources to ensure longevity. By overcoming classification system challenges and using a consistent classification system, you can increase the coverage of risks and mitigate harm from language models.
Be careful when building and maintaining a risk classification system to avoid unintended consequences of language model deployment.
Risk Taxonomy in Risk Management
Risk identification and assessment are key to managing the threats from language models.
By using a risk taxonomy, you can bucket risks into meaningful groups and develop targeted mitigation strategies. This taxonomy provides a framework to guide the organization’s risk management efforts in a structured and systematic way.
This informs decision-making and helps prioritize resources for high-priority risks.
Risk Identification and Assessment
A comprehensive risk taxonomy is key to identifying and evaluating risks in a structured and systematic way within organizations.
A risk taxonomy helps to enhance risk identification and assessment by providing a framework to bucket and analyze risks from language models.
It helps organizations identify risks in a structured way, communicate risks consistently, and inform better decision-making.
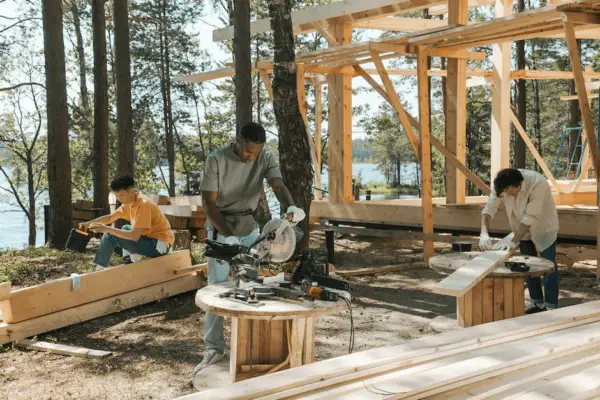
Risk Mitigation and Strategy
A robust risk taxonomy is critical to inform risk mitigation strategies and overall organizational risk management.
A well-defined risk taxonomy helps prioritize and manage risks and quickly respond to new and emerging operational risks.
By bucketing risks and understanding their impact, we can implement measures to mitigate risks and monitor them over time.
This structured approach to sound management allows for timely and informed decision-making and allocates of resources to the risks that pose the greatest threat.
By refining the risk taxonomy over time, organizations can adapt to the changing risk landscape, avoid pitfalls, and improve overall risk management.
Case Studies and Examples
Risk taxonomies have been used in real-world applications to provide a structured framework for identifying and managing risks from language models.
These examples show how bucketing risks and understanding the causal mechanisms lead to targeted mitigation strategies.
Real-World Applications and Examples
Real-world scenarios demonstrate the practicality of risk taxonomies in language models and how they protect against harm and promote ethical AI.
Risk taxonomies ensure fair patient care by detecting and mitigating biases in healthcare AI systems. They help combat misinformation to protect public health and well-being.
Risk taxonomies find and fix discriminatory outputs to promote fairness and inclusion in AI. By working, they prevent harmful content generation, such as hate speech and exclusionary language.
These examples show the importance of risk taxonomies in developing ethical guidelines and user-friendly tools to mitigate information hazards and societal risks.
Conclusion: Risk Management with a Good Taxonomy
By bucketing and understanding the risks of language models, you can address issues before they get out of hand.
This is a foundation tool for developing risk management strategies and avoiding the negative impacts of language model deployment.
Risk Taxonomy in Today’s Risk Landscape
In today’s complex risk landscape, the foundation of risk management is to have a good risk taxonomy. A good risk taxonomy is key to organizations to navigate the changing risk landscape.
It is part of a risk strategy to identify risks across internal audit, external risks, and business operations. By having a risk strategy and taxonomy in place, organizations can prioritize risk management and address threats before they happen.
This structured approach helps identify and bucket risks and allocate resources to the most critical risks. Having a good risk taxonomy is critical in today’s risk landscape to improve risk management.
FAQs
What is the Taxonomy of Risk Models?
To understand the taxonomy of risk models, consider various potential harms and dangers bucketed into discrimination, misinformation, and malicious uses.
Biased algorithm design and lack of diverse training data are the root causes of these risks, and mitigation strategies are needed.
What is the Taxonomy of AI Risks?
One must consider language models’ ethical and social dangers to understand the taxonomy of AI risks. Discrimination, misinformation, and malicious uses are the risk categories here. Frameworks show risks like biased algorithms and content amplification.
What Are the Risks of Language Models?
Risks of language models are perpetuating biases, spreading misinformation, breaching confidentiality, and enabling disinformation.
You need to address these risks to avoid discrimination, harm to health equity, trust breaches, and disruptions to public health.
What is the Risk Factor Taxonomy?
You need to understand the risk factor taxonomy for language models. It buckets risks into discrimination, misinformation, and malicious uses. Biased algorithm design and lack of diverse data are the root causes of these risks. Mitigation is bias detection and ethical guidelines.
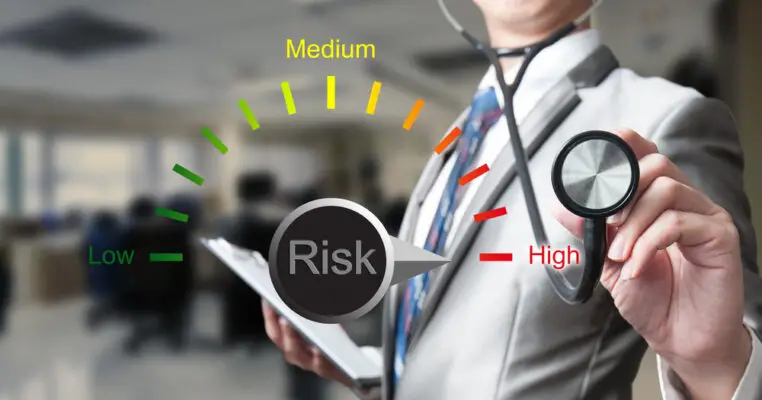
Conclusion
In summary, the Taxonomy of Risks of Language Models is a foundation to understand and mitigate the risks in development and deployment.
By addressing issues like bias, discrimination, and misinformation, organizations can manage risks proactively and use language models more ethically.
Having bias detection algorithms and ethical guidelines in place is key to avoiding unintended consequences and leveraging the benefits of these powerful tools across domains.
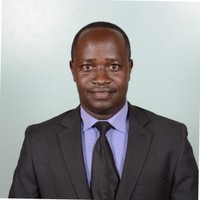
Chris Ekai is a Risk Management expert with over 10 years of experience in the field. He has a Master’s(MSc) degree in Risk Management from University of Portsmouth and is a CPA and Finance professional. He currently works as a Content Manager at Risk Publishing, writing about Enterprise Risk Management, Business Continuity Management and Project Management.