Model Risk Management (MRM) refers to managing and mitigating risks that arise from using mathematical models in business decision-making.
Mathematical models play a crucial role in various aspects of business, including financial forecasting, risk assessment, algorithmic trading, valuation, and more. While these models can be extremely powerful, they also carry risks due to potential inaccuracies or errors.
Model risk can arise from several sources, including:
Model Specification: The model may be based on incorrect or overly simplistic assumptions, leading to inaccurate results.
Parameter Estimation: The parameters used in the model may be estimated incorrectly, leading to errors in the model’s output.
Implementation Errors: There could be errors in how the model is coded or implemented, leading to incorrect results.
Usage Errors: The model may be used incorrectly or inappropriately, leading to misinterpretations or misusing the model’s results.
Model Risk Management aims to identify and mitigate these risks. This typically involves:
Model Validation: This includes verifying that a model is working as intended, that its assumptions and methodologies are sound, and that its results are accurate and reliable.
Model Governance: This involves establishing policies and procedures for the development, validation, and use of models, including documentation requirements, roles and responsibilities, and approval processes.
Model Monitoring: This involves regularly monitoring and updating models to ensure they continue to perform accurately and reliably as market conditions and business needs change.
Model Inventory Management: This involves maintaining a comprehensive inventory of all models the organization uses, including their purpose, design, owner, and validation status.
Model Risk Management ensures that models are reliable, well understood, and used appropriately and that model risk is effectively managed across the organization.
Models are essential for banks and other financial institutions to make risk management decisions, pricing strategies, and capital allocation.
However, using models also introduces new risks that must be managed effectively.
Model risk is the potential for adverse consequences resulting from errors or inadequacies in model design, implementation, validation, or application.
Despite their widespread use and importance, many financial institutions have yet to effectively develop a comprehensive approach to managing model risk.
One reason is the perceived cost and effort required to implement such a program. However, given the increasing regulatory scrutiny on model risk management and its potential impact on business operations and reputation, organizations must understand what it entails and why it is crucial for long-term success.
This article provides an overview of what model risk management means in practice and how institutions can mitigate these risks through sound policies and procedures.
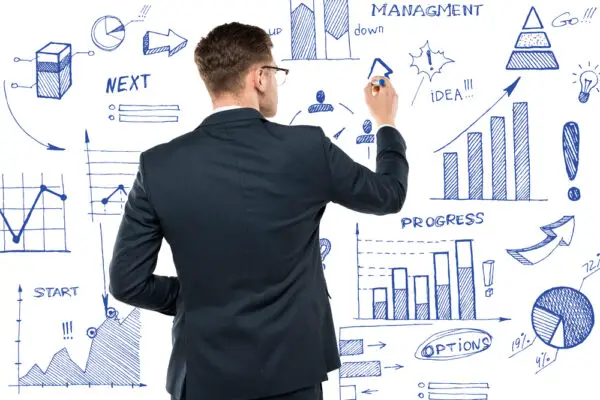
Understanding the Importance of Model Risk Management
Correctly identifying and mitigating potential weaknesses in analytical processes can give organizations increased confidence in the validity and reliability of their decision-making.
It plays a crucial role in decision-making as it helps organizations avoid errors that could lead to incorrect decisions.
Regulatory compliance is another important aspect of model risk management. Regulatory agencies require financial institutions to have robust model risk management practices to ensure they use models appropriately and do not expose themselves or others to unnecessary risks.
Proper implementation of model risk management can help organizations meet regulatory requirements while ensuring their models’ accuracy and reliability.
Organizations can make informed decisions based on accurate data by identifying potential weaknesses in analytical processes, mitigating risks, and complying with regulatory standards.
Implementing an effective model risk management program requires ongoing assessment and monitoring to ensure it remains relevant and effective.
Identifying and Assessing Risks Associated with Models
In order to navigate potential pitfalls, it is essential to thoroughly examine the factors that may impact the veracity and reliability of predictive tools. Quantitative analysis involves identifying and assessing different types of risks associated with models.
These include input data quality, model assumptions, parameter estimation errors, model selection issues, and limitations in predicting rare events.
Risk assessment is a crucial component of model risk management as it helps identify vulnerabilities in models that can lead to inaccurate results or erroneous decisions.
It involves evaluating the likelihood and potential impact of identified risks on stakeholders such as investors, regulators, customers, or employees. This process requires gathering relevant data from various sources, such as historical data, expert opinions, market trends, and best practices.
Once risks are identified and assessed, appropriate mitigating measures must be implemented to reduce their impact or likelihood. Some common strategies for managing model risk include improving data quality controls.
Implementing stress testing procedures; establishing governance structures that ensure transparency and accountability; developing contingency plans for unexpected events; conducting ongoing monitoring and review processes; and providing training programs for staff responsible for using models.
Following these steps rigorously while staying up-to-date with industry standards and best practices will help organizations manage their model risk management program effectively while minimizing exposure to potential harm.
Mitigating Model Risk through Policies and Procedures
Potential issues can be mitigated by implementing comprehensive policies and procedures that provide a framework for identifying and addressing vulnerabilities in predictive tools.
Risk appetite is an important consideration when developing these policies, as it determines the level of risk that an organization is willing to accept.
Regulatory compliance is another key factor in mitigating model risk. Organizations must adhere to regulatory standards and guidelines for model development, validation, testing, and usage.
This includes ensuring transparency in using models and providing documentation that demonstrates their accuracy and reliability. Failure to comply with regulations can result in significant financial penalties and damage an organization’s reputation.
In addition to policies and procedures, ongoing monitoring and evaluation are essential for effective model risk management. Regular reviews should be conducted to identify any changes in assumptions or market conditions that may impact model performance.
Adjustments should be made accordingly while ensuring updates are properly documented and communicated throughout the organization.
Effective mitigation strategies require a proactive approach focusing on constant improvement through monitoring, evaluation, and adjustment based on changing circumstances.
Best Practices for Model Validation and Governance
The first step towards achieving this goal is conducting thorough stress testing of models. This involves subjecting models to various scenarios with different inputs, outputs, and assumptions to determine their performance under adverse conditions.
Stress testing helps identify weaknesses in the model and enables organizations to take corrective measures before it’s too late.
In addition to stress testing, documentation requirements are critical in ensuring model validity. Documentation should be comprehensive enough for independent reviewers to understand the model’s design, development, testing methods, and results.
It should also include details about data sources used in building the model and assumptions made during its construction. Documented evidence helps demonstrate how decisions were made throughout the modeling process and provides a basis for future reviews.
Organizations must also implement appropriate governance structures that oversee all aspects of model development from inception through retirement. Model risk management committees should be established with clearly defined roles and responsibilities.
The Multiple functions include risk management, finance, audit, and compliance departments. These committees regularly review models according to pre-defined criteria, such as changes in market conditions or regulatory requirements.
Ensuring Long-Term Success through Effective Model Risk Management
Establishing a robust framework for predictive tool oversight can give organizations the foundation to instill confidence in their decision-making processes, ultimately leading to more informed and successful outcomes.
A long-term strategy is essential for effective model risk management, as it helps organizations identify potential risks that may arise from using predictive tools and develop appropriate mitigation plans.
This requires regular monitoring and updating of models, continuous training of staff on new developments in the field, and frequent review of compliance with regulatory requirements.
Risk assessment techniques are another critical component of effective model risk management.
Organizations need to thoroughly understand their models’ limitations, including areas where they may be prone to errors or biases. Adopting advanced analytics tools and technologies can help organizations detect these issues before they escalate into significant problems.
Additionally, establishing clear guidelines on how models should be used and when they should be retired can help prevent unintended consequences from flawed predictions.
It is an ongoing process that requires continuous monitoring and adaptation as new challenges arise in the rapidly evolving field of data analytics.
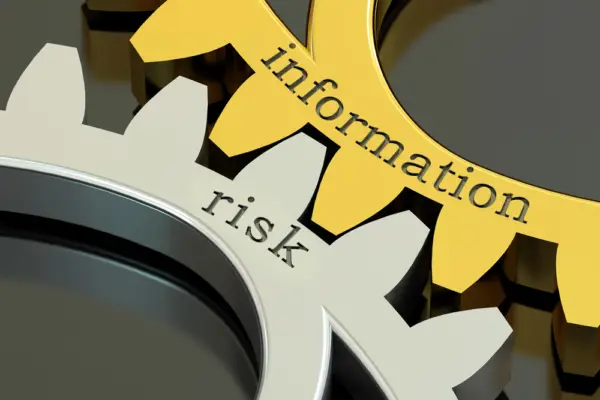
Frequently Asked Questions
What are some common types of models that require risk management?
Credit scoring and market forecasting are two standard models requiring risk management.
Financial institutions use credit scoring models to assess the creditworthiness of individuals or businesses seeking loans. These models consider various factors, such as credit history, income, and debt-to-income ratios, to determine the likelihood of loan repayment.
On the other hand, market forecasting models are used by investors to predict future market trends and make investment decisions accordingly. These models use historical data and various economic indicators to predict future trends in stock prices, interest rates, and other financial variables.
Given the significant impact that these models can have on financial decision-making processes, organizations need to implement robust model risk management practices to ensure accurate results and minimize potential risks associated with model errors or biases.
What are the consequences of not correctly managing model risk?
The consequences of not correctly managing model risk can be severe. In fact, according to a recent survey conducted by the Global Association of Risk Professionals, over 40% of financial institutions have experienced financial losses due to model failures within the past two years.
These losses can range from minor errors to significant monetary damages that impact the institution’s bottom line. Poor risk management practices can also lead to reputational damage and loss of trust among customers and investors alike.
How do regulatory requirements impact model risk management practices?
Regulatory compliance is a critical consideration in the management of model risk. To meet regulatory requirements, financial institutions must have robust policies and procedures for identifying, assessing, and monitoring model risk.
This involves conducting thorough risk assessments to identify potential issues with models and implementing appropriate controls to mitigate these risks.
In addition, institutions must ensure that all models are subject to ongoing validation and testing to maintain their accuracy and effectiveness.
Failure to comply with regulatory requirements can lead to significant consequences, including fines, reputational damage, and legal action.
What role do stakeholders play in model risk management?
Stakeholder engagement is critical in ensuring that all parties involved understand the risks associated with modeling activities.
This includes those directly involved in the modeling process, senior management, and other relevant stakeholders.
Effective stakeholder engagement also helps promote transparency and accountability, essential to any successful model risk management framework.
Ultimately, by working together to identify and address risks, stakeholders can help protect their organizations from potentially catastrophic consequences resulting from inadequate or ineffective model risk management practices.
How can technology be used to enhance model risk management processes?
In finance, technology applications have become increasingly important in enhancing model risk management processes.
The benefits of using technology include increased efficiency and accuracy in assessing and managing model risks. However, it is essential to note that there are also limitations to relying solely on technology solutions.
Best practices for implementation involve a combination of human expertise and technological tools that complement each other. Industry trends and innovations suggest that machine learning algorithms and artificial intelligence can be used to improve model validation processes.
Additionally, blockchain technology can increase transparency and accountability in model risk management practices.
As businesses continue to rely more heavily on models for decision-making, implementing effective technological solutions will be crucial for mitigating potential risks.
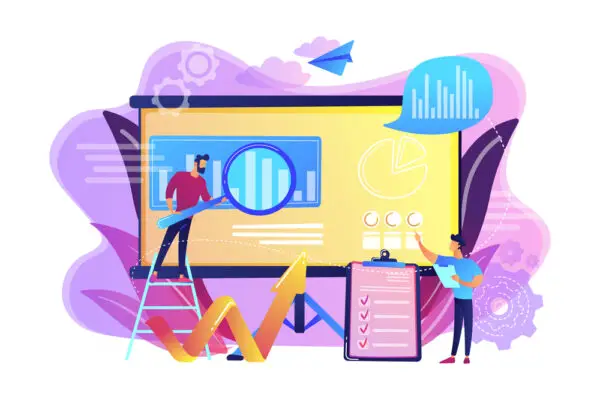
Conclusion
Model Risk Management (MRM) is a vital aspect of risk management that helps organizations mitigate the risks associated with models. MRM involves identifying, assessing, and mitigating model risks through policies and procedures to ensure long-term success.
Best practices for model validation and governance require rigorous testing and monitoring to confirm the accuracy of models.
Effective MRM requires consistent stakeholder communication, including model developers, senior management, and regulators. It is essential to implement a robust framework for managing model risk that includes regular reviews and updates as necessary.
Organizations can achieve this by adopting best practices in data collection, analysis, modeling techniques, and documentation.
Organizations can minimize potential losses by implementing effective policies and procedures for identifying and mitigating model risks while ensuring long-term success.
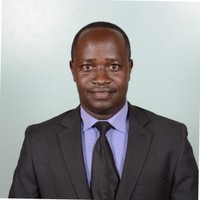
Chris Ekai is a Risk Management expert with over 10 years of experience in the field. He has a Master’s(MSc) degree in Risk Management from University of Portsmouth and is a CPA and Finance professional. He currently works as a Content Manager at Risk Publishing, writing about Enterprise Risk Management, Business Continuity Management and Project Management.