AI risk management involves the application of artificial intelligence technologies to predict, identify, and mitigate potential risks in various domains.
AI can enhance risk management by providing advanced data analysis, pattern recognition, and predictive modeling capabilities.
Here are some ways AI is used in risk management:
- Threat Intelligence Analysis: AI can analyze vast amounts of data to identify potential security threats and vulnerabilities, enabling organizations to respond to risks more quickly and effectively.
- Security Information and Event Management (SIEM): AI-powered SIEM systems can help in real-time monitoring, detection, and analysis of security events, leading to improved incident response.
- Fraud Detection: AI can be used to detect fraudulent activities by analyzing behavioral patterns and transaction data, which is particularly useful in the financial services industry.
- Workplace Risk Reduction: AI can help in monitoring workplace environments to identify safety hazards and ensure compliance with health and safety regulations.
- Data Protection: AI can assist in protecting sensitive information by identifying and classifying data, monitoring access, and detecting potential data breaches.
- Operational Risks: AI can predict and manage risks related to business operations, supply chains, and market fluctuations by analyzing various internal and external factors.
Implementing AI in risk management, however, comes with its own set of challenges, such as ensuring the quality of data, dealing with the black-box nature of some AI models, and maintaining the transparency and explainability of AI-driven decisions.
For a more in-depth understanding of the benefits and challenges of using AI in risk management, you can refer to the article “AI in Risk Management: Top Benefits and Challenges Explained.”
Additionally, the “AI Risk Management Framework” developed by NIST provides guidelines for managing risks associated with AI systems to individuals, organizations, and society.
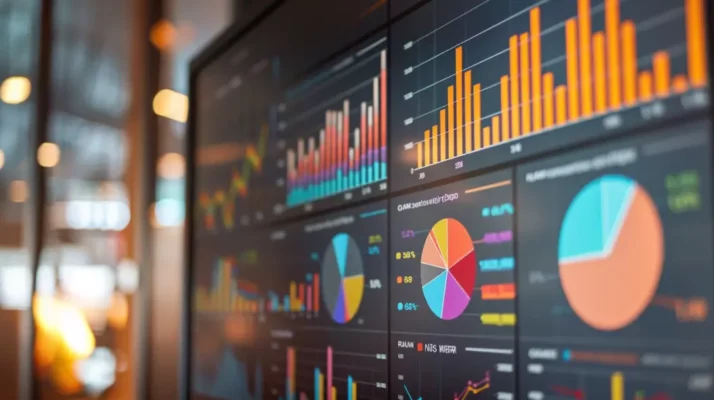
AI risk management is essential to address potential threats in artificial intelligence systems. By using methods like risk matrices, scenario analysis, and system audits, risks can be identified and assessed effectively.
Mitigation strategies such as robust testing protocols, data privacy measures, and continuous monitoring practices are vital in managing these risks.
Ethical considerations, bias detection, and regulatory compliance play significant roles in ensuring the responsible development and operation of AI systems.
Implementing structured approaches and transparent governance frameworks are key to mitigating vulnerabilities and fostering trust in AI technologies.
Discover more insights by exploring the strategies and challenges associated with AI risk management.
Key Takeaways
- Risk matrices categorize risks by likelihood and impact.
- Robust testing protocols identify and rectify errors.
- Real-time tracking detects anomalies in AI system performance.
- Transparency and explainability enhance trust in AI algorithms.
- GDPR and HIPAA ensure ethical and secure AI operations.
Importance of AI Risk Management
AI risk management plays an important role in safeguarding organizations against potential threats posed by artificial intelligence technologies.
By employing efficient risk identification methods, businesses can proactively pinpoint vulnerabilities and address them before they escalate.
Mitigation strategies and continuous monitoring practices further enhance the resilience of AI systems, ensuring peak performance and security.
Risk Identification Methods
When considering the importance of AI risk management, one of the pivotal aspects to address is the effective identification of potential risks through robust methods.
Utilizing structured approaches to identify and assess risks associated with AI systems is essential in mitigating potential harm or negative consequences. Various risk identification methods can be employed to thoroughly analyze and understand the potential threats that AI systems may pose.
One such method is the use of risk matrices, which categorize risks based on their likelihood and impact. Additionally, scenario analysis allows for the exploration of different situations that may lead to risks.
Another method involves conducting thorough audits of AI systems to uncover vulnerabilities and potential areas of concern.
Risk Identification Methods | Description | Example |
---|---|---|
Risk Matrices | Categorize risks based on likelihood and impact to prioritize and address them effectively | High likelihood of data breach with severe impact |
Scenario Analysis | Explore different situations that may lead to risks and understand their potential implications | Simulating a cyber-attack to assess vulnerabilities |
System Audits | Conduct thorough reviews of AI systems to identify vulnerabilities and areas requiring attention | Examining code for potential security loopholes |
Mitigation Strategies Overview
Exploring effective strategies for mitigating risks associated with artificial intelligence systems is essential in ensuring the overall success and safety of AI implementations.
Mitigation strategies aim to address potential vulnerabilities and threats that could arise from the deployment of AI technologies.
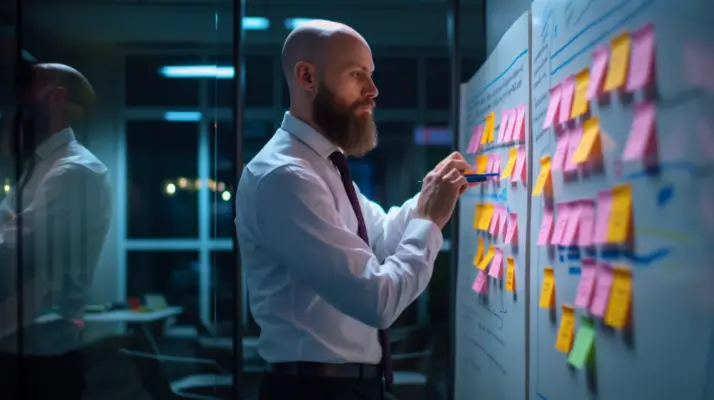
These strategies may include:
- Robust testing protocols to identify and rectify errors.
- Implementing strict data privacy measures to protect sensitive information.
- Incorporating transparency and explainability into AI algorithms to enhance trust.
- Establishing clear governance frameworks to monitor and regulate AI systems.
Continuous Monitoring Practices
Effective management of AI risks hinges on the implementation of robust continuous monitoring practices to proactively identify and address potential vulnerabilities and threats in artificial intelligence systems.
Continuous monitoring involves real-time tracking of AI system performance, data inputs, and outputs to detect anomalies or deviations from expected behavior.
By continuously analyzing system operations, organizations can swiftly respond to emerging risks, such as data breaches, algorithm biases, or model drift.
This proactive approach enables prompt corrective actions, ensuring the reliability, security, and ethical compliance of AI technologies. Implementing thorough monitoring mechanisms allows for the early detection of issues, facilitating timely interventions to mitigate risks and uphold the integrity of AI systems in an ever-evolving digital landscape.
Key Challenges in AI Risk
Addressing the challenges in AI risk involves maneuvering through ethical dilemmas arising from the use of artificial intelligence.
Developing effective bias detection techniques to guarantee fair outcomes is another crucial aspect. Enhancing transparency in algorithms to promote accountability and trust is equally important.
These key points underscore the intricate landscape surrounding AI risk management and highlight the critical areas that require attention and innovative solutions to mitigate potential risks effectively.
By understanding and proactively addressing these challenges, organizations can foster responsible AI deployment and minimize adverse impacts on individuals and society.
Ethical Dilemmas in AI
In the domain of AI risk management, ethical dilemmas pose intricate challenges that demand careful consideration and proactive strategies.
One of the key ethical dilemmas in AI revolves around issues of bias, privacy, accountability, and transparency. Addressing these dilemmas requires a nuanced understanding of the ethical implications of AI systems and the potential risks they pose to individuals and society as a whole.
By implementing robust ethical frameworks and guidelines, organizations can navigate these challenges and guarantee that AI technologies are developed and deployed responsibly.
The table below provides a snapshot of some common ethical dilemmas in AI:
Ethical Dilemma | Description |
---|---|
Bias in AI | Unintended discrimination in AI algorithms |
Privacy Concerns | Protection of user data and information privacy |
Accountability | Determining responsibility for AI decisions |
Transparency | Understanding how AI systems reach conclusions |
Bias Detection Techniques
Detecting bias in AI systems presents a critical challenge in the domain of AI risk management. Necessitating advanced techniques to guarantee fairness and equity in algorithmic decision-making.
Bias detection techniques involve analyzing datasets for disparities in outcomes across different demographic groups. Methods such as statistical parity, disparate impact analysis, and fairness constraints are employed to identify and mitigate biases.
Additionally, researchers are developing tools like AI audit frameworks and bias detection algorithms to enhance transparency and accountability in AI systems.
By implementing these techniques, organizations can diminish the potential harm caused by biased algorithms and ensure that AI technologies operate ethically and equitably in various applications, from hiring processes to predictive policing.
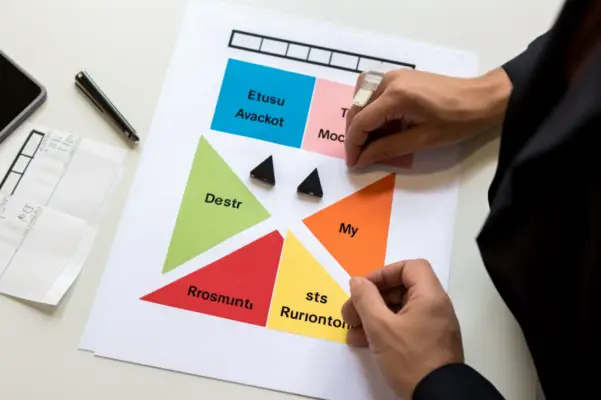
Transparency in Algorithms
Ensuring transparency in algorithms remains a paramount challenge within the domain of AI risk management, critical for fostering trust and accountability in algorithmic decision-making processes.
Transparency involves making the inner workings of algorithms understandable to non-technical stakeholders, enabling them to comprehend how decisions are reached.
Lack of transparency can lead to unintended consequences, erode trust, and hinder the identification of biases or errors.
Various methods can enhance transparency, such as providing clear explanations of how algorithms work, disclosing data sources, and offering avenues for redress in case of errors.
Transparency not only aids in identifying and addressing potential risks but also empowers users to make informed decisions regarding algorithmic outcomes, promoting accountability and ethical use of AI technologies.
Considerations for AI Risk
In the domain of AI risk, several important considerations come to the fore:
Ethical AI practices are pivotal to ensuring that artificial intelligence systems operate in a manner that aligns with societal values and norms.
Data privacy concerns highlight the significance of safeguarding individuals’ personal information from unauthorized access or misuse.
Regulatory compliance measures play a vital role in establishing frameworks that govern the development and deployment of AI technologies, ensuring adherence to legal requirements and industry standards.
Ethical AI Practices
Implementing ethical AI practices is essential in mitigating potential risks associated with artificial intelligence technology.
By ensuring that AI systems are developed and deployed with a focus on ethical considerations, organizations can build trust with users, minimize biases, and promote transparency. Below is a table highlighting key aspects of ethical AI practices:
Ethical AI Practices | Description | Importance |
---|---|---|
Transparency | Providing clear explanations of how AI systems make decisions and operate. | Helps build trust and accountability. |
Fairness | Ensuring that AI algorithms are unbiased and treat all individuals fairly. | Reduces discrimination and promotes inclusivity. |
Accountability | Holding individuals and organizations responsible for the outcomes of AI systems. | Encourages ethical behavior and oversight. |
Data Privacy Concerns
With the increasing integration of artificial intelligence (AI) technology into various aspects of society, safeguarding data privacy has become a critical concern in mitigating potential risks associated with AI applications.
Data privacy concerns arise due to the vast amount of personal data collected, stored, and processed by AI systems.
Issues such as unauthorized access, data breaches, and misuse of data can lead to severe consequences for individuals and organizations.
To address these concerns, implementing robust data protection measures, such as encryption, access controls, and anonymization techniques, is vital.
Additionally, transparency about data collection practices and obtaining informed consent from users are essential for maintaining trust and upholding ethical standards in AI development and deployment.
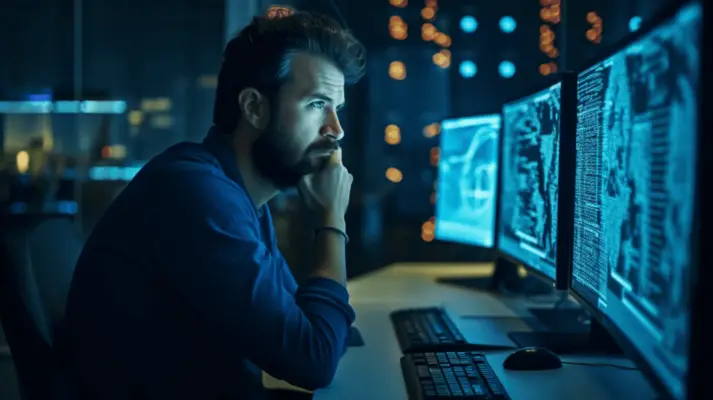
Regulatory Compliance Measures
The adherence to regulatory compliance measures is paramount in effectively managing the risks associated with artificial intelligence (AI) implementation. Regulations such as the General Data Protection Regulation (GDPR) and the Health Insurance Portability and Accountability Act (HIPAA) are crafted to guarantee that AI systems operate ethically, transparently, and securely.
Compliance with these measures helps organizations mitigate the potential legal, financial, and reputational consequences of AI-related incidents. By following regulatory guidelines, businesses can foster trust among consumers, protect sensitive data, and uphold ethical standards in their AI practices.
Regular audits, documentation of processes, and ongoing training for employees are essential components of maintaining regulatory compliance in the rapidly evolving landscape of AI technologies.
Strategies for Mitigating AI Risks
To effectively mitigate AI risks, organizations must prioritize ethical AI development. This involves ensuring that AI systems align with moral and societal values. Transparency in algorithms is also crucial. It enables stakeholders to understand how decisions are made and holds AI systems accountable.
Continuous monitoring systems play an essential role in detecting and addressing any potential risks or biases that may arise in AI operations.
Ethical AI Development
Strategies for mitigating AI risks through ethical development involve establishing clear guidelines and frameworks that prioritize responsible decision-making in the design and deployment of artificial intelligence systems.
By incorporating ethical considerations into the development process, potential harms and biases can be identified and addressed proactively.
This approach aims to guarantee that AI systems act in ways that align with societal values and norms, promoting trust and acceptance among users. Below is a table highlighting key strategies for ethical AI development:
Ethical AI Development Strategies | Description | Benefits |
---|---|---|
Data Privacy Compliance | Ensuring data collection and usage adhere to regulations | Enhanced user trust |
Bias Detection and Mitigation | Identifying and correcting biases in AI algorithms | Improved fairness |
Explainability of AI Decisions | Making AI processes transparent and understandable | Increased accountability |
User Consent and Control | Allowing users to understand and control AI interactions | Empowerment and autonomy |
Ethical Oversight Mechanisms | Implementing mechanisms for ethical reviews and audits | Ensuring ethical compliance |
Transparency in Algorithms
By fostering transparency in algorithms, organizations can enhance accountability and trust in artificial intelligence systems.
Transparency in algorithms involves making the decision-making processes of AI systems understandable and traceable. This transparency allows for the identification of biases, errors, or unethical practices within the algorithms.
Organizations can achieve transparency by documenting and disclosing the data sources, methodologies, and assumptions used in developing AI algorithms.
Additionally, creating standardized frameworks for explaining AI decisions can increase transparency and facilitate external scrutiny.
By promoting transparency, organizations not only mitigate the risks associated with opaque AI systems but also build credibility with stakeholders and users.
Transparent algorithms contribute to a more ethical and responsible deployment of artificial intelligence technologies.
Continuous Monitoring Systems
Continuous monitoring systems play an essential role in detecting and addressing potential risks associated with the deployment of artificial intelligence technologies.
These systems continuously assess AI algorithms, data inputs, and outcomes to identify any anomalies or biases that may emerge over time.
By implementing real-time monitoring tools, organizations can promptly respond to issues such as algorithm drift, data poisoning, or model degradation.
Through regular audits and performance evaluations, continuous monitoring enhances transparency and accountability in AI systems, ensuring that they operate ethically and effectively.
Additionally, these systems enable proactive risk management by alerting stakeholders to deviations from expected behavior, allowing for timely interventions and adjustments to mitigate potential harms before they escalate.
Essentially, continuous monitoring is a cornerstone of responsible AI governance and risk mitigation strategies.
Implementing Effective AI Risk Management
To effectively manage AI risks, organizations need to implement robust risk assessment strategies and mitigation techniques.
Risk assessment strategies involve identifying potential risks associated with AI systems, analyzing their likelihood and impact, and prioritizing them for mitigation efforts.
Mitigation techniques focus on implementing controls, monitoring systems, and response plans to reduce the likelihood and impact of identified risks.
Risk Assessment Strategies
What are the key strategies for effectively implementing AI risk management through thorough risk assessment methods?
In the domain of AI risk management, employing robust risk assessment strategies is crucial. One essential approach is to conduct exhaustive risk assessments that involve identifying potential risks associated with AI systems.
This includes evaluating the impact of these risks on various aspects such as data security, privacy, and ethical considerations.
Additionally, leveraging advanced technologies like machine learning algorithms can aid in analyzing vast amounts of data to proactively identify potential risks.
Furthermore, collaborating with multidisciplinary teams comprising AI experts, ethicists, and legal professionals can provide diverse perspectives for a more holistic risk assessment process.
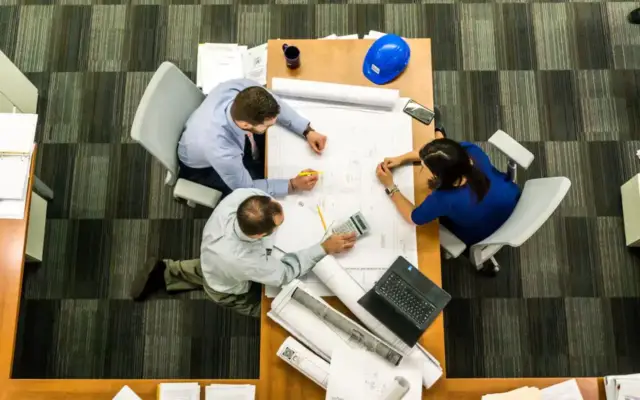
Mitigation Techniques
To effectively implement AI risk management and mitigate potential risks, organizations must deploy targeted mitigation techniques that address identified vulnerabilities within their AI systems.
These techniques encompass a range of strategies, including rigorous testing protocols to detect and rectify flaws, implementing robust cybersecurity measures to protect against malicious attacks, and ensuring transparency and explainability in AI decision-making processes.
Additionally, organizations can utilize techniques such as data anonymization to safeguard sensitive information, regularly updating AI algorithms to adapt to evolving threats, and incorporating human oversight to intervene in critical situations.
By integrating these mitigation techniques into their AI risk management framework, organizations can enhance the resilience and reliability of their AI systems, ultimately minimizing the likelihood of adverse outcomes.
Frequently Asked Questions
How Can AI Risk Management Benefit Businesses Beyond Just Avoiding Negative Outcomes?
Businesses can leverage AI risk management to not only prevent negative outcomes but also enhance decision-making, improve operational efficiency, identify new opportunities, and gain a competitive edge.
It serves as a strategic asset for overall business success.
What Are the Ethical Considerations Involved in AI Risk Management?
Ethical considerations in AI risk management encompass issues like transparency, fairness, accountability, and bias mitigation.
Ensuring that AI systems operate ethically is pivotal to building trust, protecting privacy, and upholding moral standards in technology development and deployment.
How Can Organizations Effectively Measure the Success of Their AI Risk Management Strategies?
Organizations can measure the success of their AI risk management strategies by evaluating key performance indicators, such as the reduction in AI-related incidents, the level of compliance with regulatory requirements, and the overall impact on business operations and reputation.
Are There Any Regulatory Requirements or Guidelines Related to AI Risk Management That Businesses Should Be Aware Of?
Businesses should stay informed about regulatory requirements and guidelines related to risk management to guarantee compliance.
Understanding these rules is essential for maintaining ethical standards, mitigating risks, and fostering trust with stakeholders.
How Can Businesses Ensure That Their AI Risk Management Strategies Are Adaptable to New and Emerging Technologies in the Future?
Businesses can guarantee adaptability by staying informed of industry trends, fostering a culture of innovation, investing in ongoing training for employees, leveraging agile methodologies, and regularly reviewing and updating their AI risk management strategies to align with emerging technologies.
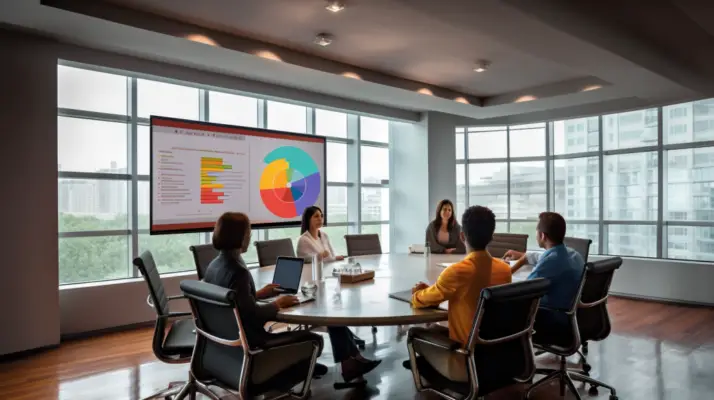
Conclusion
To sum up, effective AI risk management is vital in safeguarding against potential dangers and ensuring the responsible development and deployment of artificial intelligence technologies.
By understanding the key challenges, considering various factors, and implementing appropriate strategies, organizations can mitigate risks associated with AI.
It is essential to prioritize risk management in AI to foster trust, transparency, and accountability in the rapidly evolving landscape of artificial intelligence.
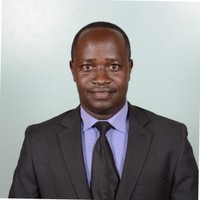
Chris Ekai is a Risk Management expert with over 10 years of experience in the field. He has a Master’s(MSc) degree in Risk Management from University of Portsmouth and is a CPA and Finance professional. He currently works as a Content Manager at Risk Publishing, writing about Enterprise Risk Management, Business Continuity Management and Project Management.